Last week we hosted a free Kubeflow and MLOps workshop presented by Kubeflow Community Product manager Josh Bottum. In this blog post we’ll recap some highlights from the workshop, plus give a summary of the Q&A. Ok, let’s dig in.
First, thanks for voting for your favorite charity!
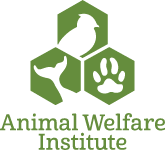
With the unprecedented circumstances facing our global community, Arrikto is looking for even more ways to contribute. With this in mind, we thought that in lieu of swag we could give attendees the opportunity to vote for their favorite charity and help guide our monthly donation to charitable causes. The charity that won this month’s workshop voting was Animal Welfare Institute. We are pleased to be making a donation of $250 to them on behalf of the Kubeflow community. Again, thanks to all of you who attended and voted!
What topics were covered in the workshop?
- How to install Kubeflow via MiniKF locally or on a public cloud
- Take a snapshot of your notebook
- Clone the snapshot to recreate the exact same environment
- Create a pipeline starting from a Jupyter notebook
- Go back in time using Rok. Reproduce a step of the pipeline and view it from inside your notebook
- Create an HP Tuning (Katib) experiment starting from your notebook
- Serve a model from inside your notebook by creating a KFServing server
- An overview of what’s new in Kubeflow 1.4
What did I miss?
Here’s a short teaser from the 45 minute workshop where Josh walks us through a Kubeflow Pipeline execution graph with extra emphasis on how to create and work with data and artifact snapshots at every step.
Install MiniKF
In the workshop Josh discussed how MiniKF is the easiest way to get started with Kubeflow on the platform of your choice (AWS, GCP or locally). He also talked about the basic mechanics of installing MiniKF.
Here’s the links:
Hands-on Tutorials
Although during the workshop, Josh focused primarily on the examples shown in tutorial #3 (which makes heavy use of the Open Vaccine Covid-19 example), we highly recommend to also try out tutorial #4 which does a great job of walking you through all the steps you’ll need to master, when bringing together all the Kubeflow components to turn your models into pipelines. You can get started with these hands-on, practical tutorials by following these links:
- Tutorial 1: An End-to-End ML Workflow: From Notebook to Kubeflow Pipelines with MiniKF & Kale
- Tutorial 2: Build An End-to-End ML Workflow: From Notebook to HP Tuning to Kubeflow Pipelines with Kale
- Tutorial 3: Build an ML pipeline with hyperparameter tuning and serve the model starting from a notebook
- Tutorial 4: Build an AutoML workflow starting from a notebook
Need help?
Join the Kubeflow Community on Slack and make sure to add the #minikf channel to your workspace. The #minikf channel is your best resource for immediate technical assistance regarding all things MiniKF!
Missed the Nov 18 workshop?
If you were unable to join us last week but would still like to attend a workshop in the future you can sign up for the next workshop happening on Dec 23.
Links to Resources
For those that attended the workshop, here’s the resource links you need to replicate the exercises:
- Kubeflow Community Resources all in one place
- Install MiniKF
- Kubeflow Tutorials
- Find and join a local Kubeflow Meetup
- Upcoming training and certification preparation courses
Announcing Arrikto Academy
I should also point out that the workshops are a feeder for the more advanced offerings of “Arrikto Academy”, which is Arrikto’s new skills-based Kubeflow education initiative. If you’d like to explore additional training options aimed at intermediate to advanced users of Kubeflow, you should definitely check out Arrikto Academy! (You can also read the announcement blog here.)
In fact, we’ve recently released our first two courses – Kale 101 and Katib 101 – both of which focus on the necessary Day 1 Fundamentals that all data scientists need to be successful with the Kubeflow ecosystem. The courses are capped off with self-graded labs to ensure skills are being developed and retained.
Q&A from the workshop
Below is a summary of some of the questions that popped into the Q&A box during the workshop. [Edited for readability and brevity.]
What is the difference between Kubeflow and MLflow?
Kubeflow is an end-to-end MLOps platform that facilitates the entire workflow from experimentation, to training, to hyperparameter optimization, all the way to model deployment. MLflow is a library for metadata tracking for artifacts and provides a model registry as well. Note that users have integrated Kubeflow with MLflow in the past. Finally, Kubeflow has recently introduced a backend for artifact tracking called MLMD (Machine Learning Metadata).
In the Kubeflow demo I see that it is running on GCP. Google offers Vertex AI that also uses Kubeflow underneath the covers. Any idea what the difference is between the two?
Vertex AI makes use of the Pipelines component of Kubeflow itself to orchestrate pipelining. Note that Vertex also includes closed source, managed services from Google. It should not be thought of as a managed Kubeflow deployment.
Can Kubeflow be integrated with Pycaret?
We don’t see a reason why someone couldn’t use PyCaret with Kubeflow. Please note that many components within the Kubeflow platform are framework agnostic, that’s the beauty of open source!
The demo being shown is on Google Cloud, what is the estimated monthly cost for running MiniKF?
It utilizes just a single VM instance which will be roughly $330 per month. You can view the detailed pricing and discounts available in the GCP marketplace.
Are there any tutorials on how to use the Kale add-on?
A few links to pursue:
- Kale project on GitHub
- Kubeflow tutorials which make use of Kale
- Video: A Complete Introduction to Kubeflow
- Video: Getting Started with the Kale SDK
Where can I find the roadmap for the Kubeflow 1.5 version?
Look for an update on what’s coming in the 1.5 release by checking the official roadmap page on GitHub.
Does Kubeflow support Microsoft’s DeepSpeed AI?
We haven’t yet seen DeepSpeed discussed within the Kubelfow community, but it shouldn’t be impossible to integrate it. Please note that many components within the Kubeflow platform are framework agnostic, so it’s easy to integrate new components to the whole Kubeflow ecosystem.
What is the difference between KFServing and Kubeflow Pipelines (KFP)?
KFServing is the model deployment/inference component of Kubeflow. KFP is the Kubeflow Pipelines component of Kubeflow.
What’s Next?
- Join the Kubeflow community on Slack and take a moment to introduce yourself!
- Quickly get Kubeflow up and running by getting started with MiniKF
- Contact us to schedule a private Kubeflow and MiniKF workshop for your team.