This week we hosted a free Kaggle Titanic Disaster Machine Learning workshop. In this blog post we’ll recap some highlights from the workshop, plus give a summary of the Q&A. Ok, let’s dig in.
First, thanks for voting for your favorite charity!
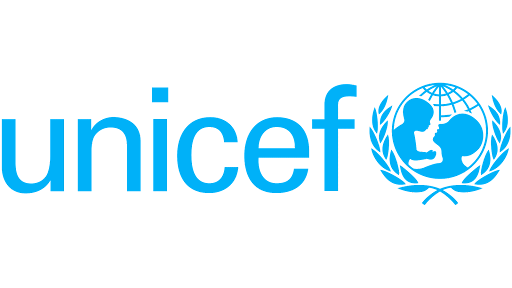
With the unprecedented circumstances facing our global community, Arrikto is looking for even more ways to contribute. With this in mind, we thought that in lieu of swag we could give workshop attendees the opportunity to vote for their favorite charity and help guide our monthly donation to charitable causes. The charity that won this workshop’s voting was UNICEF. Also known as the United Nations Children’s Fund, UNICEF is a United Nations agency responsible for providing humanitarian and developmental aid to children worldwide. The agency is among the most widespread and recognizable social welfare organizations in the world, with a presence in 192 countries and territories. We are pleased to be making a donation of $200 to them on behalf of the workshop attendees. Again, thanks to all of you who attended and voted!
What topics were covered in the workshop?
- Overview of Kubeflow
- Installing Kubeflow
- About the Titanic Disaster Example
- Deploying a Notebook Server
- Getting the Titanic Disaster Example Up and Running
- Exploring the Notebook
- Deploying and Running a Pipeline
- Examining the Results
What did I miss?
Here’s a short teaser from the 45 minute workshop where I walked folks through how to get the Titanic Disaster notebook up and running on a Kubeflow cluster.
Want to see more? Here’s the YouTube playlist of all the demos featured in the workshop, plus the complete playback.
Install MiniKF
In the workshop I showed how MiniKF is the easiest way to get started with Kubeflow on the platform of your choice (AWS or GCP). Want to try it for yourself? Here’s the links:
Try the Titanic Disaster Tutorial for Yourself
If you’d like to work through the Titanic Disaster notebook yourself, check out the guided tutorial on Arrikto Academy.
Need help?
Join the Kubeflow Community on Slack and make sure to add the #minikf channel to your workspace. The #minikf channel is your best resource for immediate technical assistance regarding all things MiniKF!
Missed the Feb 9 workshop?
If you were unable to join us this week, but would still like to attend a workshop in the future, register for one of these upcoming workshops.
Q&A from the workshop
Below is a summary of some of the questions that popped into the Q&A box during the workshop. [Edited for readability and brevity.]
What is the relation between Kubeflow and Arrikto?
On the community side, Arrikto has been contributing to the Kubeflow project since version 0.4. Along with participating in various working groups (WGs), they are also members of the release management teams for versions 1.3, 1.4 & 1.5. Finally, they help organize 12 Kubeflow/MLOps Meetups and deliver free weekly workshops, training, and certification programs.
Since Kubeflow runs on Kubernetes, can it run on public clouds?
Yes! Kubeflow can theoretically run anywhere Kubernetes can. For example, you have the following packaged distribution options on the following clouds:
AWS
- Kubeflow on AWS, by AWS
- Enterprise Kubeflow on EKS, by Arrikto
- MiniKF, by Arrikto
Google Cloud
- Kubeflow on Google Cloud, by Google
- Enterprise Kubeflow on GKE, by Arrikto
- MiniKF, by Arrikto
Azure
- Kubeflow on Azure, by Microsoft
- Enterprise Kubeflow on AKS, by Arrikto
I am planning on deploying Kubeflow on GCP. Are there step by step instructions for how to get MiniKF up and running on GCP similar to the AWS instructions shown?
Yes. Check out: https://www.arrikto.com/minikf-on-gcp-installation-instructions/
I’d like to install Kubeflow on AKS. Can I do this using the manifests advanced option?
At Arrikto we offer a packaged distribution of Kubeflow 1.4 for AKS. You can also check out the Kubeflow on Azure docs on how to get up and running, with what currently appears to be an older Kubeflow 1.2 version. You can also try to install Kubeflow on your own from the latest Kubeflow 1.4 manifests.
Can we have a pipeline that calls big data processing jobs using Spark inside of Kubeflow Pipelines?
Yes. It is a common practice to call Spark jobs from within Kubeflow. In fact, we’ve had two talks on this topic at previous Meetups. Check them out:
- Comparing Apache Spark on Kubernetes to Kubeflow
- Orchestrating Apache Spark with Kubeflow on Kubernetes
Is it possible to add the Kale extension to an existing Kubeflow Notebook Server that is running on GCP? If so, are their instructions?
We are working on a way to do this. Stayed tuned for some exciting developments in regards to Kale.
Can MiniKF run locally, on a laptop?
You can do this via Vagrant and VirtualBox. However, it does require significant resources to be available, 32GB of RAM, 2 CPUS and 40 GBs of storage at least. Because of this, we don’t recommend local installs if you have GCP or AWS available as deployment platforms. Additionally, you’ll need to account for resources to actually run your experiments. But, it can be done.